Peer-Reviewed A.I. in Vet Med

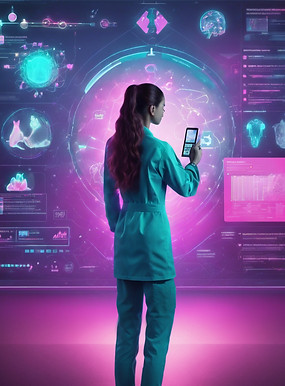
Important - before you start reading the papers:
​
Just as peer review ensures reliability in medicine, it’s essential for artificial intelligence models to also undergo similar scrutiny before they are integrated into practice. With that said, be aware that the current landscape of artificial intelligence regulation is sparse and fragmented--even for some of the models that are making it into our practices. Because of this, like any other facet of medicine, it's important to understand the science behind what you're doing.
​
Here's a quick introduction to how to evaluate where these A.I. models stand in development.
​
There are 3 main stages of proper A.I. model development: training, validation, and deployment. It is very important to understand that there is no legal requirement for A.I. models to have undergone any complete portion of this process to make it into your practice. Yeah, that's scary! Hopefully regulations will come along soon to protect consumers of these models (but still allow for innovation). However, for now, you need to do your due diligence to understand the equipment you're using, the basis for how it works, and--wait--has it even been proven to work in the real world?
​
How to evaluate these papers to understand what A.I. development stage they describe:
Stage 1: Training
-
What happens in this stage?
-
The model learns patterns and relationships from pre-selected data, often tested on a subset to ensure it generalizes well.
-
-
Results of this stage:
-
Preliminary accuracy is assessed - yeah, it looks like it could work??
-
-
How to identify this stage when reading these papers:
-
Often focuses on the initial dataset used to teach the model, details of how it was built, and the algorithms employed
-
-
Data results unique to this stage:
-
Training Loss (without mentioning unseen data or validation results)
-
Training Accuracy (without comparing it to validation accuracy)
-
Epoch-Based Loss Reduction (with no mention is made of generalization to new data)
-
Precision, Recall, and F1-Score (if no validation or test set is mentioned)
-
Graphs: Loss and Accuracy Curves (If validation curves are absent)
-
Context Clues:
-
No mention of a validation set
-
Explicitly states results are reported on the training data​
-
Hyperparameters (which are often adjusted in the training stage) are mentioned - learning rate, batch size, or optimizer type
-
Overfitting Signs (meaning the model is trained too specifically to the training data and cannot generalize to new data accurately) - very high training accuracy, very low training loss (without comparing to validation results)
-
-
-
Concerns:
-
Stage 1 A.I. models are not ready for real-world deployment without explicit consent of those participating. At the end of Stage 1, these models have only proven that they can work well in a lab setting on the data that the researchers have given them. They have not yet proven real-world accuracy.
-
​
Stage 2: Validation
-
What happens in this stage?
-
The model is tested on new data to evaluate its ability to generalize beyond the training dataset. This stage ensures the model’s patterns work reliably on new data. This often happens in controlled versions of real-world environments. (So, the participants still know they're guinea pigs.)
-
-
Results of this stage:
-
The "lab" model that worked in Stage 1 is put to the test to see if it will still function when the chaos of the real world (sort of) is thrown at it—and yeah, it works!​
-
-
How to identify this stage when reading these papers:​
-
The paper discusses testing the model on unseen data or mentions a validation set separate from the training data.
-
Results focus on evaluating the model’s generalizability rather than improving its performance on the training set.
-
May include comparisons between training and validation performance.​
-
-
Data results unique to this stage:​
-
Validation Loss: Measures how well the model predicts on unseen data. If validation loss is stable or decreasing, the model may generalize well.
-
Validation Accuracy: Percentage of correct predictions on the validation set.
-
Performance Metrics on Validation Data: Precision, Recall, F1-Score, ROC-AUC
-
Validation Curves: Graphs showing validation loss and accuracy over time, often plotted alongside training curves for comparison.​
-
-
Context Clues:​
-
The paper describes a validation set as distinct from the training data.
-
Discussion of overfitting or generalization concerns, often highlighting gaps between training and validation performance.
-
Metrics are explicitly labeled as validation results or compared to training metrics.
-
Mentions techniques to improve generalization, such as early stopping or regularization.​
-
-
Concerns:
-
Stage 2 models are still not fully ready for real-world deployment. Validation demonstrates the model’s ability to perform well on reserved data, but it does not guarantee success in the craziness that is your daily life in vet med. There is one more stage to go to be certain you're dealing with an A.I. model that has well-proven accuracy in real world environments.​
-
​
Stage 3: Deployment
-
What happens in this stage?
-
The model is integrated into real-world applications and tested on entirely new, diverse, and dynamic datasets. This stage evaluates how the AI performs in practical, real-world scenarios outside the controlled lab environment.
-
-
Results of this stage:
-
The model’s effectiveness in real-world use is assessed—can it hold up to the ridiculousness that is vet med?
-
-
How to identify this stage when reading these papers:
-
The paper describes testing the model in real-world settings or on datasets collected from live environments, such as clinics or user-generated data.
-
Results include field-testing metrics, feedback from users, or descriptions of real-world challenges the model faced.
-
Mentions of deployment in specific applications (ex: diagnostics, inventory management, or client interactions).
-
-
Data results unique to this stage:
-
Real-World Accuracy: The model’s performance metrics (ex: accuracy, precision, recall) are calculated on entirely new, unselected data.​
-
Performance Metrics in Operational Contexts: Precision, Recall, F1-Score, ROC-AUC when applied in specific scenarios (ex: diagnosing a condition in real patients).
-
Time-to-prediction
-
Resource efficiency metrics
-
Error Analysis in Real-World Use: Common causes of misclassification or failure in practical settings.
-
Example: “The model struggled with data variability, such as incomplete patient histories.”
-
-
Feedback from End Users: Qualitative or quantitative feedback from veterinarians or stakeholders using the deployed AI.
-
Example: “92% of users found the AI tool helpful for client communication.”
-
-
-
Context Clues:
-
Discusses specific real-world applications, such as live clinic settings, practice tools, or operational improvements in practices.
-
Mentions challenges faced during deployment, such as variability in data quality, user adoption, or scalability.
-
Results reflect conditions that are difficult to replicate in a lab setting, such as unpredictable user inputs (meaning, people do all kinds of crazy things) or a wider array of medical cases or signalments.
-
Descriptions of ongoing monitoring or updates, like retraining the model with new data or handling feedback.
-
-
Concerns:
-
Models that make it through Stage 3 are the real deal. This real-world deployment puts it to the test, revealing unexpected weaknesses in robustness, bias, or usability. With that said, ongoing monitoring is essential to ensure consistent performance, identify errors quickly, and retrain the model as data inevitably evolves over time.
-
​
Okay, now you've earned your papers:
​
Peer-Reviewed A.I. Papers in Vet Med
Cardiology
2024
Valente, C., Wodzinski, M., Guglielmini, C., Poser, H., Chiavegato, D., Zotti, A., Venturini, R., & Banzato, T. (2024). Development of an artificial intelligence-based algorithm for predicting the severity of myxomatous mitral valve disease from thoracic radiographs by using two grading systems. Research in Veterinary Science, 178, 105377- https://doi.org/10.1016/j.rvsc.2024.105377
2020
​Li, S., Wang, Z., Visser, L. C., Wisner, E. R., & Cheng, H. (2020). Pilot study: Application of artificial intelligence for detecting left atrial enlargement on canine thoracic radiographs. Veterinary Radiology & Ultrasound, 61(6), 611–618. https://doi.org/10.1111/vru.12901
Internal Medicine
(Large Animal)
2022
Liu, K., Liu, L., Tai, M., Ding, Q., Yao, W., & Shen, M. (2022). Light from heat lamps affects sow behaviour and piglet salivary melatonin levels. Animal : an international journal of animal bioscience, 16(6), 100534. https://doi.org/10.1016/j.animal.2022.100534
2022
Teixeira, V. A., Lana, A. M. Q., Bresolin, T., Tomich, T. R., Souza, G. M., Furlong, J., Rodrigues, J. P. P., Coelho, S. G., Gonçalves, L. C., Silveira, J. A. G., Ferreira, L. D., Facury Filho, E. J., Campos, M. M., Dorea, J. R. R., & Pereira, L. G. R. (2022). Using rumination and activity data for early detection of anaplasmosis disease in dairy heifer calves. Journal of dairy science, 105(5), 4421–4433. https://doi.org/10.3168/jds.2021-20952
Internal Medicine
(Small Animal)
2023
​Patkar, S., Mannheimer, J., Harmon, S. A., Ramirez, C. J., Mazcko, C. N., Choyke, P. L., Brown, G. T., Turkbey, B., LeBlanc, A. K., & Beck, J. A. (2024). Large-Scale Comparative Analysis of Canine and Human Osteosarcomas Uncovers Conserved Clinically Relevant Tumor Microenvironment Subtypes. Clinical cancer research : an official journal of the American Association for Cancer Research, 30(24), 5630–5642. https://doi.org/10.1158/1078-0432.CCR-24-1854
Oncology
2024
Patkar, S., Mannheimer, J., Harmon, S. A., Ramirez, C. J., Mazcko, C. N., Choyke, P. L., Brown, G. T., Turkbey, B., LeBlanc, A. K., & Beck, J. A. (2024). Large-Scale Comparative Analysis of Canine and Human Osteosarcomas Uncovers Conserved Clinically Relevant Tumor Microenvironment Subtypes. Clinical cancer research : an official journal of the American Association for Cancer Research, 30(24), 5630–5642. https://doi.org/10.1158/1078-0432.CCR-24-1854
2023
Wang, S., Pang, X., de Keyzer, F., Feng, Y., Swinnen, J. V., Yu, J., & Ni, Y. (2023). AI-based MRI auto-segmentation of brain tumor in rodents, a multicenter study. Acta neuropathologica communications, 11(1), 11. https://doi.org/10.1186/s40478-023-01509-w
Ophthalmology
2011
Wang, Q., Grozdanic, S. D., Harper, M. M., Hamouche, N., Kecova, H., Lazic, T., & Yu, C. (2011). Exploring Raman spectroscopy for the evaluation of glaucomatous retinal changes. Journal of biomedical optics, 16(10), 107006. https://doi.org/10.1117/1.3642010
Parasitology
2024
Nagamori, Y., Scimeca, R., Hall-Sedlak, R., Blagburn, B., Starkey, L. A., Bowman, D. D., Lucio-Forster, A., Little, S. E., Cree, T., Loenser, M., Larson, B. S., Penn, C., Rhodes, A., & Goldstein, R. (2024). Multicenter evaluation of the Vetscan Imagyst system using Ocus 40 and EasyScan One scanners to detect gastrointestinal parasites in feces of dogs and cats. Journal of veterinary diagnostic investigation : official publication of the American Association of Veterinary Laboratory Diagnosticians, Inc, 36(1), 32–40. https://doi.org/10.1177/10406387231216185
2024
Steuer, A., Fritzler, J., Boggan, S., Daniel, I., Cowles, B., Penn, C., Goldstein, R., & Lin, D. (2024). Validation of Vetscan Imagyst®, a diagnostic test utilizing an artificial intelligence deep learning algorithm, for detecting strongyles and Parascaris spp. in equine fecal samples. Parasites & vectors, 17(1), 465. https://doi.org/10.1186/s13071-024-06525-w
Pathology
2024
Pihlman, H., Linden, J., Paakinaho, K., Hannula, M., Morelius, M., Manninen, M., Laitinen-Vapaavuori, O., & Keränen, P. (2024). Long-term comparison of two β-TCP/PLCL composite scaffolds in rabbit calvarial defects. Journal of applied biomaterials & functional materials, 22, 22808000241299587. https://doi.org/10.1177/22808000241299587
2006
​Price, J. R., Aykac, D., & Wall, J. (2006). A 3D level sets method for segmenting the mouse spleen and follicles in volumetric microCT images. Conference proceedings : ... Annual International Conference of the IEEE Engineering in Medicine and Biology Society. IEEE Engineering in Medicine and Biology Society. Annual Conference, 2006, 2332–2336. https://doi.org/10.1109/IEMBS.2006.260127
Radiology
2023
Wang, S., Pang, X., de Keyzer, F., Feng, Y., Swinnen, J. V., Yu, J., & Ni, Y. (2023). AI-based MRI auto-segmentation of brain tumor in rodents, a multicenter study. Acta neuropathologica communications, 11(1), 11. https://doi.org/10.1186/s40478-023-01509-w
2020
Boissady, E., de La Comble, A., Zhu, X., & Hespel, A. M. (2020). Artificial intelligence evaluating primary thoracic lesions has an overall lower error rate compared to veterinarians or veterinarians in conjunction with the artificial intelligence. Veterinary radiology & ultrasound : the official journal of the American College of Veterinary Radiology and the International Veterinary Radiology Association, 61(6), 619–627. https://doi.org/10.1111/vru.12912
2008
Li, X., Yankeelov, T. E., Peterson, T. E., Gore, J. C., & Dawant, B. M. (2008). Automatic nonrigid registration of whole body CT mice images. Medical physics, 35(4), 1507–1520. https://doi.org/10.1118/1.2889758
*Not technically AI, but laying the groundwork
2022
Adrien-Maxence, H., Emilie, B., Alois, C., Michelle, A., Kate, A., Mylene, A., David, B., Marie, S., Jason, F., Eric, G., Séamus, H., Kevin, K., Alison, L., Megan, M., Hester, M., Jaime, R. J., Zhu, X., Micaela, Z., & Federica, M. (2022). Comparison of error rates between four pretrained DenseNet convolutional neural network models and 13 board-certified veterinary radiologists when evaluating 15 labels of canine thoracic radiographs. Veterinary radiology & ultrasound : the official journal of the American College of Veterinary Radiology and the International Veterinary Radiology Association, 63(4), 456–468. https://doi.org/10.1111/vru.13069
2013
McEvoy, F. J., & Amigo, J. M. (2013). Using machine learning to classify image features from canine pelvic radiographs: evaluation of partial least squares discriminant analysis and artificial neural network models. Veterinary radiology & ultrasound : the official journal of the American College of Veterinary Radiology and the International Veterinary Radiology Association, 54(2), 122–126. https://doi.org/10.1111/vru.12003
2008
Maroy, R., Boisgard, R., Comtat, C., Frouin, V., Cathier, P., Duchesnay, E., Dollé, F., Nielsen, P. E., Trébossen, R., & Tavitian, B. (2008). Segmentation of rodent whole-body dynamic PET images: an unsupervised method based on voxel dynamics. IEEE transactions on medical imaging, 27(3), 342–354. https://doi.org/10.1109/TMI.2007.905106
*Not technically AI, but laying the groundwork
Theriogenology
1992